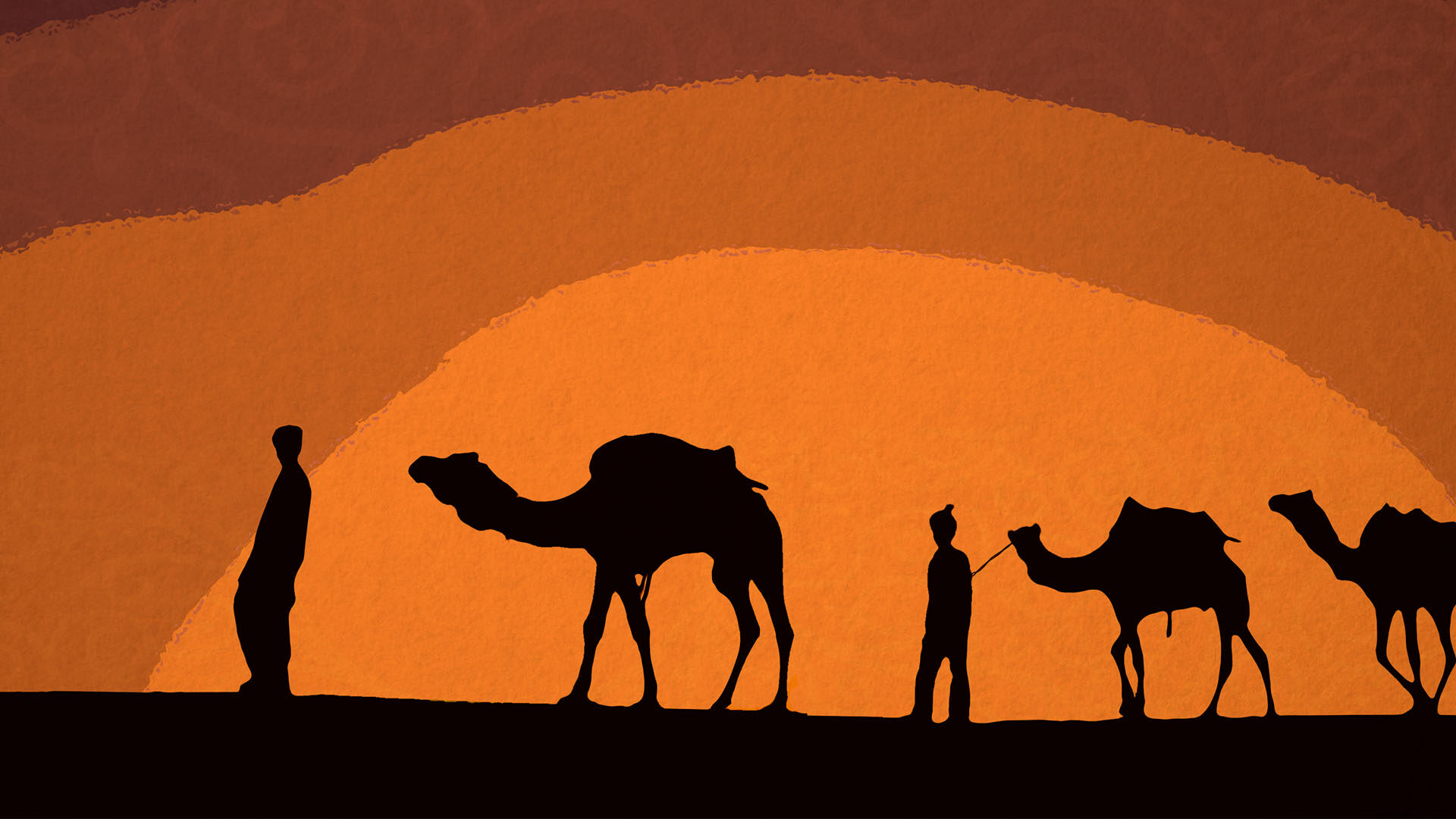
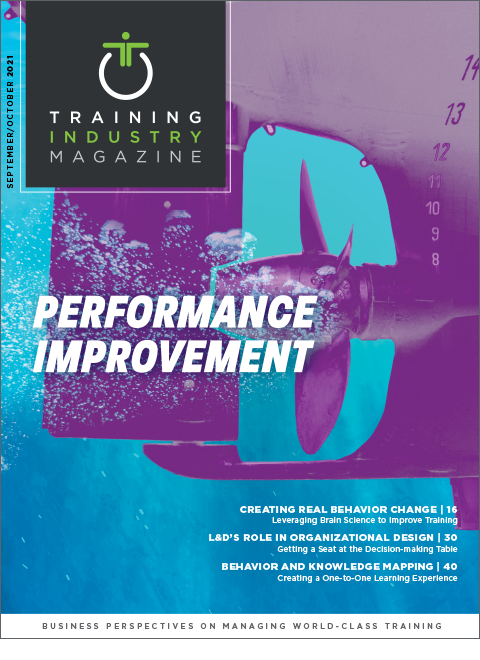
Published in Sept/Oct 2021
For the majority of human history, to learn a trade, an apprentice worked side-by-side with an employer, learning directly from him (or her) and then practicing the craft until they perfected it. Employers didn’t just give the apprentice a multiple-choice test to evaluate their readiness. Rather, they watched how an apprentice behaved and performed, and then provided feedback — ensuring the apprentice learned the necessary skills and could confidently apply them.
Why One-to-One Learning?
The days of the traditional apprenticeship are long gone. But, as study after study has shown, this level of personalization and one-to-one interaction is hard to beat when it comes to its efficacy and efficiency. Additionally, studies also show that one-to-one learning promotes greater learning, increased motivation, and also enhances persistence, retention and degree attainment.
Why is it so effective? One reason is that in the one-to-one scenario, the coach isn’t just paying attention to what a learner knows. They are also observing and adjusting based on learner behavior. For example, a coach is gaining actionable insights by evaluating what a learner does when they haven’t mastered a skill: Do they go back and source the skill? Do they attempt to problem solve? Do they lose confidence? Based on a learner’s behavior, the coach can offer individualized remediation and practical recommendations for improvement. They can also optimize the level of difficulty of the learning, making for a much more effective and efficient learning experience.
Historically, one-to-one learning hasn’t been widely adopted because it’s not scalable and often not logistically possible.
However, we have entered a new era of possibilities. Through a combination of learning science, artificial intelligence and machine learning, UI/UX, and data analytics, the most advanced digital learning platforms are leveraging new insights — derived from behavior and knowledge mapping — to emulate the personalized, one-to-one learning experience at scale. And in doing so, they are tapping into the other 50 percent of the learning equation.
What is behavior and knowledge mapping?
Behavior and knowledge mapping leverages machine learning algorithms to monitor learner behavior (the choices that they make within a learning platform), along with learning performance, to dynamically adjust content, hints, feedback, recommendations and “nudges.” Through behavior and knowledge mapping, adaptive learning platforms can effectively mimic and scale the one-to-one learning experience — dramatically improving the amount of content that’s mastered, remembered and accurately applied, with confidence.
With a combination of technology, UX and data science, this behavioral input becomes a critical part of the adaptive learning puzzle. And it’s important to note that a system’s AI capabilities are only as good as the accuracy, depth and breadth of the input it gets fed. When, as historically has been the case, systems rely only on knowledge as the core data point, they’re not providing the AI with the whole picture. As a result, they are less capable and less efficient.
But combining these two data sets — performance and behavior — drives dynamic adaptivity with greater precision and accuracy, and allows learning platforms to operate like a one-to-one coach at scale:
- Intensively personalizing the learning experience in real time to increase application-level mastery and boost learner confidence.
- Dialing content for optimal challenge, meaning it’s the appropriate difficulty level for the “sweet spot” of learning — not too easy, not too hard.
- Flagging any skills gaps and encouraging deliberate practice and self-remediation (with support).
- Offering insights into behavioral drivers, including the attitudes, aptitudes and eventual output of each employee.
And because behavior and knowledge mapping does not rely on or need any personal identifiable information (PII) from the learner, the resulting recommendations and real-time content alterations are completely unbiased.
How Behavior and Knowledge Mapping Works
It all begins with choices.
Research has demonstrated that when people have autonomy over their learning experience, they pay better attention, they’re more engaged, and they remember the training more accurately and for longer periods of time. And these choices — or behaviors — not only increase learner engagement, but also increase an adaptive system’s ability to get to know and understand the learner and better serve them. This includes providing an adaptive system’s AI with the data it needs to dial in the learning “sweet spot,” or optimal challenge, for each individual. Optimal challenge is when the level of content difficulty is just above someone’s current capability, which pushes him or her to achieve greater learning gains and retain them long-term. Coupled with deliberate, purposeful practice, learning science has shown repeatedly that this is the most efficient and effective approach to learning and retention.
Choices within the learning experience might include allowing learners to:
- Choose how they want to engage with content (e.g., read it, watch a video, engage in a practice session with Q&A, etc.).
- Select how they want to receive feedback — immediately, on occasion or not at all.
- Respond to or ignore prompts reminding them to revisit/source content where they demonstrate weak performance.
Each choice that a learner makes (along with the patterns of clicks that lead up the respective choice) yields a new behavioral data point, allowing an adaptive learning platform to map, analyze and adjust to the needs of the individual learner more efficiently and effectively. These data points accumulate as a learner moves through a system — making choice after choice. And in the most advanced, data-science-driven, adaptive systems, they can be analyzed in real time by AI to generate an increasingly comprehensive picture of each learner’s aptitude, attitude and needs.
Armed with behavior and knowledge mapping, adaptive learning programs can also amalgamate the behavioral data of individuals into a group view that can predict bottom-line outcomes like productivity, performance, revenue and even culture. That’s right, this data can tell organizations if their people are dialed in, or just attempting to “fake it til they make it.” And because behavior and knowledge mapping is AI-powered, its predictions and recommendations get smarter and smarter with every learner interaction.
Behavior and Knowledge Mapping in Action
Behavior and knowledge mapping provides a wealth of advantages for both the learner and the organization. Here are some examples of behavior and knowledge mapping in action:
Example 1: A More Effective Learning Experience
One of the keys to one-to-one learning is that it’s interactive, dynamic and tailored to the unique needs of the individual. With behavior and knowledge mapping, adaptive systems can deliver something very similar, providing learners with:
- Their optimal challenge — meaning the content is challenging and engaging, to get the learner into and maintain their “learning flow.”
- More effective and timely learning “nudges” based on knowledge and behavioral input.
- Perfectly timed memory boosters to address the Forgetting Curve.
Behavior and knowledge mapping can also give an organization a much better understanding of who might be struggling, where (which skills and learning objectives) and why, with behavioral insights that are relevant and actionable.
Example 2: Proficiency with Efficiency
Most adaptive platforms simply focus on efficiency, with proficiency being secondary. Because behavior and knowledge mapping can deliver learning that’s hyper-targeted to the needs of the individual, learners are able to progress much more quickly through the content while the system objectively measures proficiency, in parallel.
Behavior and knowledge mapping has effectively flipped the old way of learning (where time was fixed and proficiency was the variable), to now having proficiency fixed and time being the variable. Learners maximize time by quickly moving past what they already know and focusing on the content they have yet to learn, with behavior and knowledge mapping directing them back to specific portions of the learning that need more attention and delivering relevant memory boosters. This process can help learners get back to work faster, and fully prepares them with the knowledge and confidence they need to succeed.
Example 3: Predicting Application of Skills
Will learners remember what they learned in training? Will they be able to apply it once they’re back on the job? Behavior and knowledge mapping data can predict the answers to these questions by evaluating things like:
- If learners can demonstrate mastery of skills by answering assessments correctly via deliberate, purposeful practice.
- If learners review content for incorrect answers.
- How successful their practice attempts are and how quickly they improve over time.
This behavior and knowledge mapping data can also be extrapolated organization-wide to form a picture of the entire workforce’s engagement, the perceived relevance of training goals and the effectiveness of content.
Conclusion
Behavior and knowledge mapping is a technology breakthrough with multiple advantages for the learner and business. It’s like working out with a personal trainer who really gets to know you. The trainer not only knows that you can bench press 200 pounds (which you might not know), but also knows that you like to sit on the couch and eat chips – and who, in knowing both what you’re physically capable of and how you behave, can prescribe a personalized workout and nutrition plan to keep you motivated and help you achieve your goals.
In the scenario above, traditional digital learning would only know how much you could bench press. And when you didn’t reach your goals, the system would be in the dark as to why. That’s because digital learning platforms have never before been able to measure the full equation of learning — knowledge and behavior — that provides a total picture. The behavior part has always been missing.
Once in place, behavior and knowledge mapping can help organizations deliver more effective learning experiences, reduced training times, predictions about on-the-job training application and improved learner engagement. It can also help organizations evaluate culture, identify the next generation of leaders and get ahead of costly mistakes before they happen.